I have posted on this blog why robots or AI should dream, but considering some of the plausible scenarios of that ability in humans I’ve come to question the issue. I don’t know how many times I’ve had the experience of waking from a dream and seeing some object like a cup or tool or even a person and as I reach out or even walk over to approach the imagined person they just vanish from thin air. What if a person can be in a state where not all of their cognitive circuits have awakened or better yet interpret the episodic events happening as a dream but they are actually are not. There is a condition known as parasomnia and somnambulism where sleep-walking-like states of individuals become violent, here is a very good paper on the subject. When researching the neural correlates of dreaming I found that REM sleep has activations that are similar to wakefulness! The image below shows what areas of the brain increase in activity and decrease as well during REM sleep.
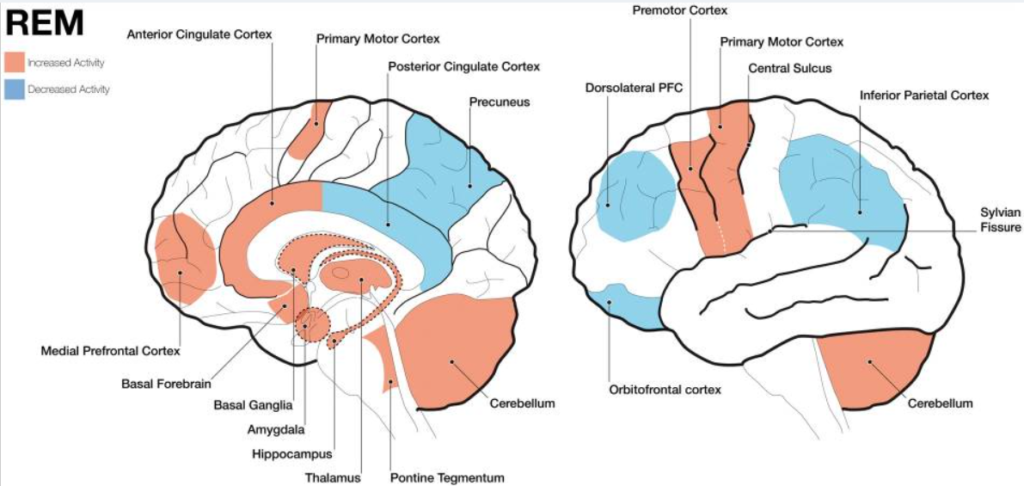
There is another phenomenon called Dream-Reality confusion which can align with psychotic symptoms! But I don’t think all dream-reality confusion derives from a disorder. As I mentioned earlier there could be cognitive circuits that are placed into a condition where the brain is in a dream state, and the person hasn’t fully awakened. Where actions that otherwise would be inhibited or corrected by such circuits don’t and the person acts out a ridiculous action(s) that could be harmful to themselves or others. This could explain how mass shootings happen. Imagine acting out by shooting people at random because of some paranoid fear or anger that your brain would normally prevent such an action but those circuits are shut down as if in a dream. Then imagine that those circuits eventually do turn back on and you are now fully cognizant of what you’ve done and realized there’s no way to turn things around, you’re confused as to how you could have even committed such an act and then take the quick way out and kill yourself!
I then realized a danger for an AGI to have the ability to dream. Because such an AI would have many computational components and they would operate asynchronously and in parallel ensuring that the AGI doesn’t become ambulatory while dreaming could get tricky and have similar problems distinguishing dreams from reality, which then could become very dangerous. Think of a bodyguard or a military soldier bot that needs to optimize or fine-tune its defense strategies, it engages in its dream state killing anyone or thing that could be a threat or even some kind of companion bot that is working out some conflict or reprimand that it experienced. Those kinds of scenarios can have violent behaviors and the bot acts them out in dreams but would never do so in its wakeful state, at least if it knows it’s awake.
It is believed that dreams in animals inclusive of humans serve to optimize and update memories. But how does that process end up as narratives as we experience in dreams? I’ve thought about how memory updates should be handled by an AGI using a time chunking approach. Below is a diagram of a time chunking implementation I’m using and here’s a link that explains it:
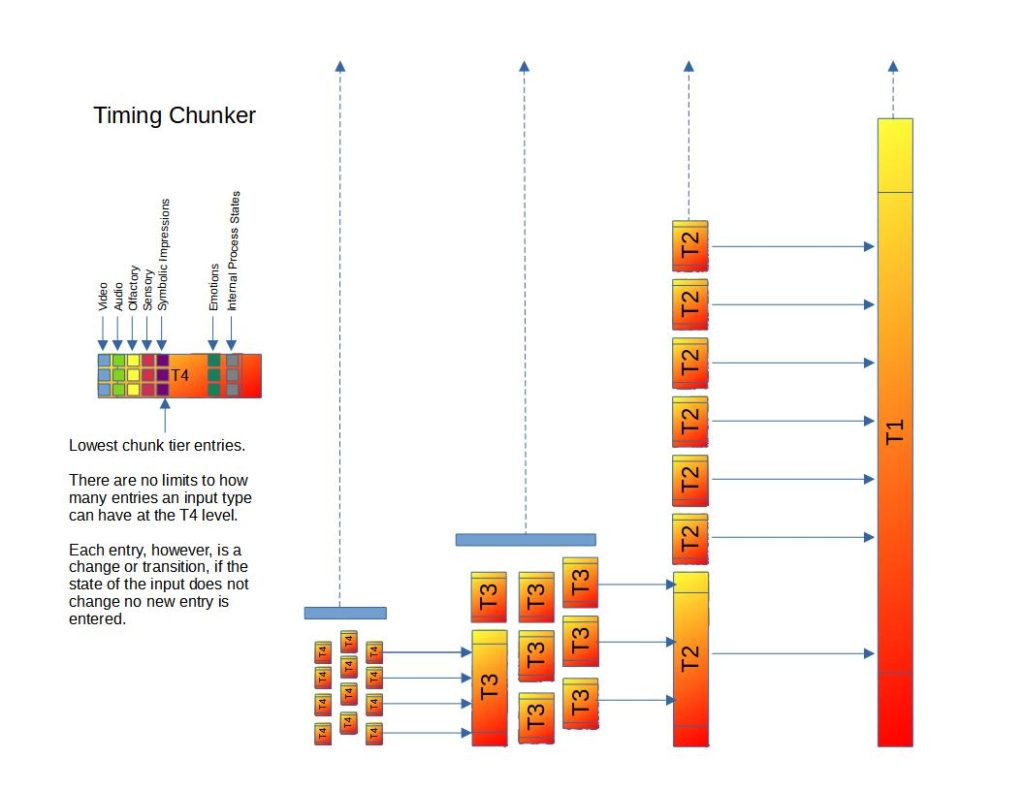
The actual updating of the memory, whether it’s an ANN or symbolic process, or a simple function does not create the narrative alone. I’m thinking of using event triggers of long-term memory updates that post to the time chunker. Those events then affect processes that would interpret the memory updated. This causes processes to use the newly updated information that will associate to ideas that create narratives. This is a good way to integrate the memory updates from dreaming into other memories. This makes sense for human brains as well, where the modified information is posted to the hippocampus and motivates other cortical components to use the now optimal memories in a simulation that tests its efficacy. So updated memories have similar effects on dream narratives from impetuses such as external sounds, indigestion, etc.
Below is an AGI model designed to have characteristics that allow for human-like decision-making. Where choices are arbitrated by emotional states that are a sense of qualia. Emotions and hormonal signaling is done with vector representations of chemistries found in biology. The diagram depicts rhythmic hormonal influences, in an animal body those hormones are distributed through the bloodstream, in this model such a distribution is done through a message sink that various software components can listen to. This is what allows for staging such an AGI to place itself in modes that will bias decision-making. This approach can also place the AGI in a dream mode that signals components like the “Situational Awareness”, “Action Sequencer”, and “Motor Control Unit” to modes to inhibit motor functions.
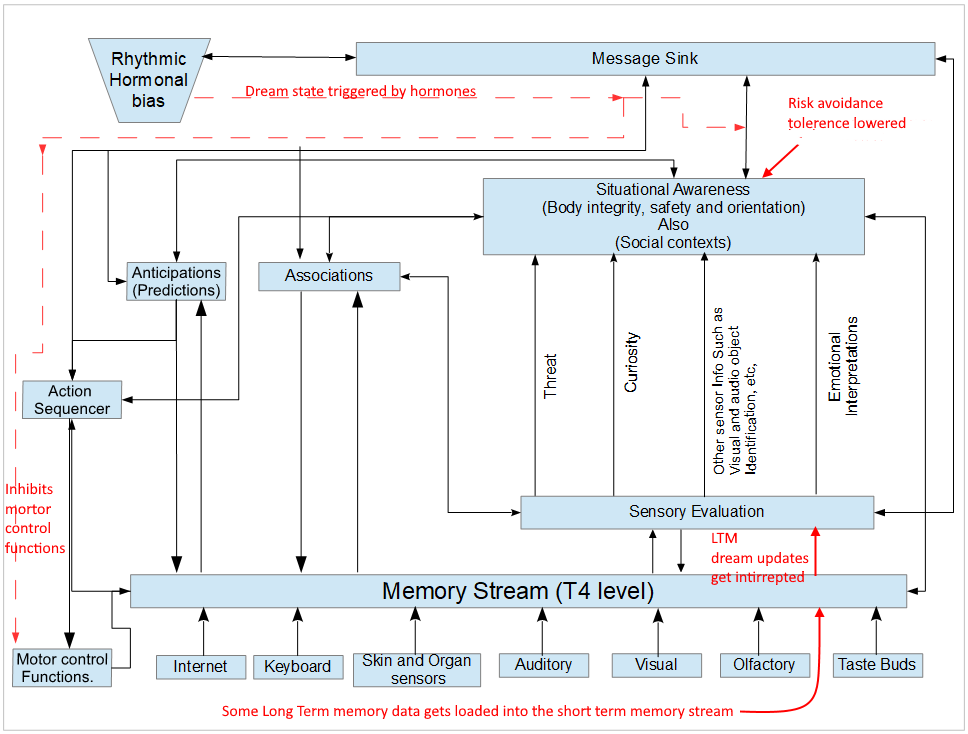
Notice that in a dream state risk avoidance and/or inhibitions are lowered in order to allow for subject matters that would otherwise be avoided or whose emotional states are very well regulated to be experienced without such regulation in a dream narrative. As long as everything works correctly in terms of inhibiting the bot’s motor controls no danger of acting out will happen, but if for whatever reason it doesn’t because the bot is in a more risk-tolerant mode safety precautions for such situations will not engage. This bot can suffer from dream-reality confusion.
This begs the question of should a robot dream considering that such a stage or state could be dangerous? There may be a need to set standards as to how to ensure such issues are addressed inclusive, perhaps, of certifications of robots that meet those standards to prevent dream-reality confusion.
I highly recommend reading the links I’ve placed in this post, you won’t be disappointed.